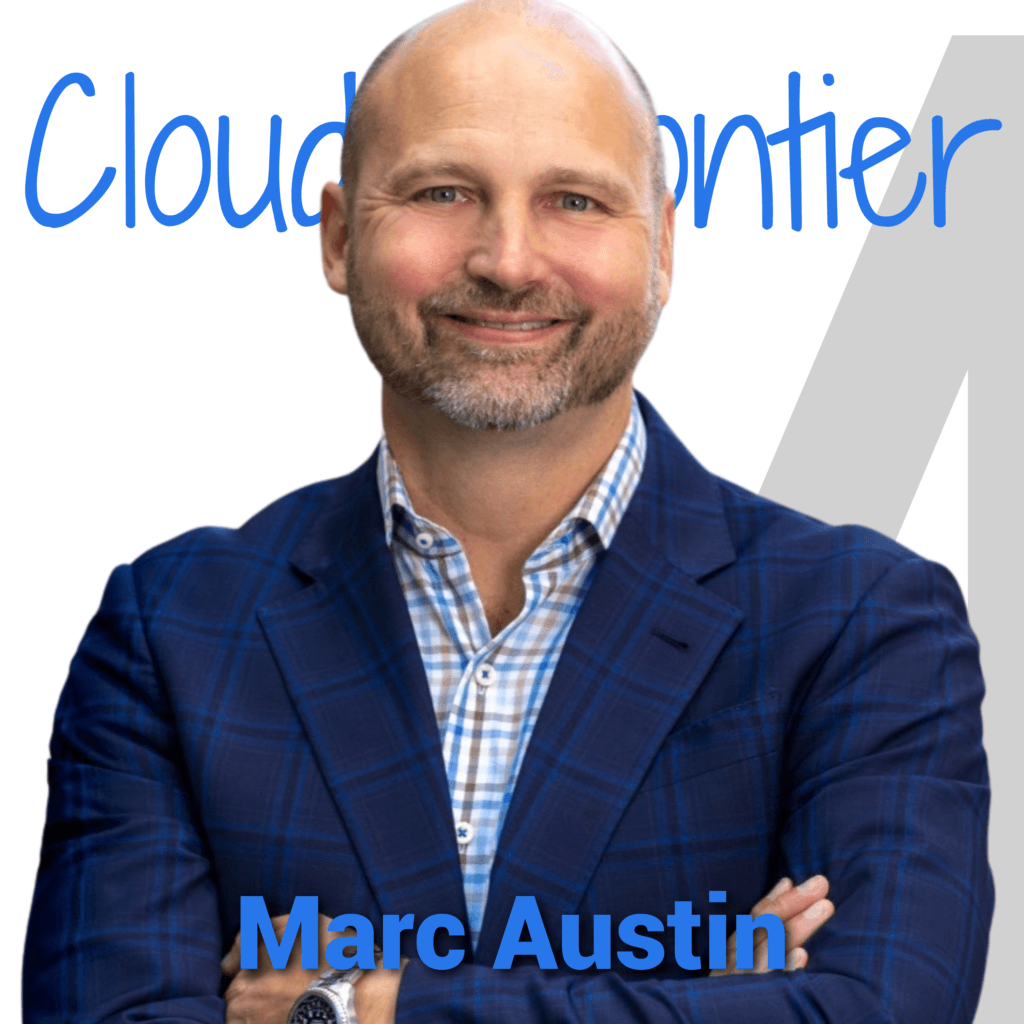
Introduction:
In this episode of the Cloud Frontier Podcast, Marc Austin, CEO and co-founder of Hedgehog, explores network infrastructure for AI. He covers the need for high-performance, cost-effective AI networks similar to AWS, Azure, and Google Cloud. Discover how Hedgehog democratizes AI networking through open-source innovation.
This interview is part of the simplyblock Cloud Frontier Podcast, available on Youtube, Spotify, iTunes/Apple Podcasts, and our show site.
Key Takeaways
What Infrastructure is Needed for AI Workloads?
AI workloads need scalable, high-performance infrastructure, especially for networking and GPUs. Marc explains how hyperscalers like AWS, Azure, and Google Cloud set the standard for AI networks. Hedgehog seeks to match this with open-source networking software. As a result, it enables efficient AI workloads without the high costs of public cloud services.
How does AI Change Cloud Infrastructure Design?
AI drives big changes in cloud infrastructure, particularly through distributed cloud models. AI inference often requires edge computing, deploying models in settings like vehicles or factories. This need spurs the development of flexible infrastructure that operates seamlessly across public, private, and edge clouds.
What is the Role of GPUs in AI Cloud Networks?
GPUs are crucial for AI workloads, especially for training and inference. Marc discusses how Luminar, a leader in autonomous vehicle tech, chose private cloud infrastructure for efficient GPU use. By using private GPUs, they avoided public cloud costs, recovering their investment within six months compared to a 36-month AWS commitment.
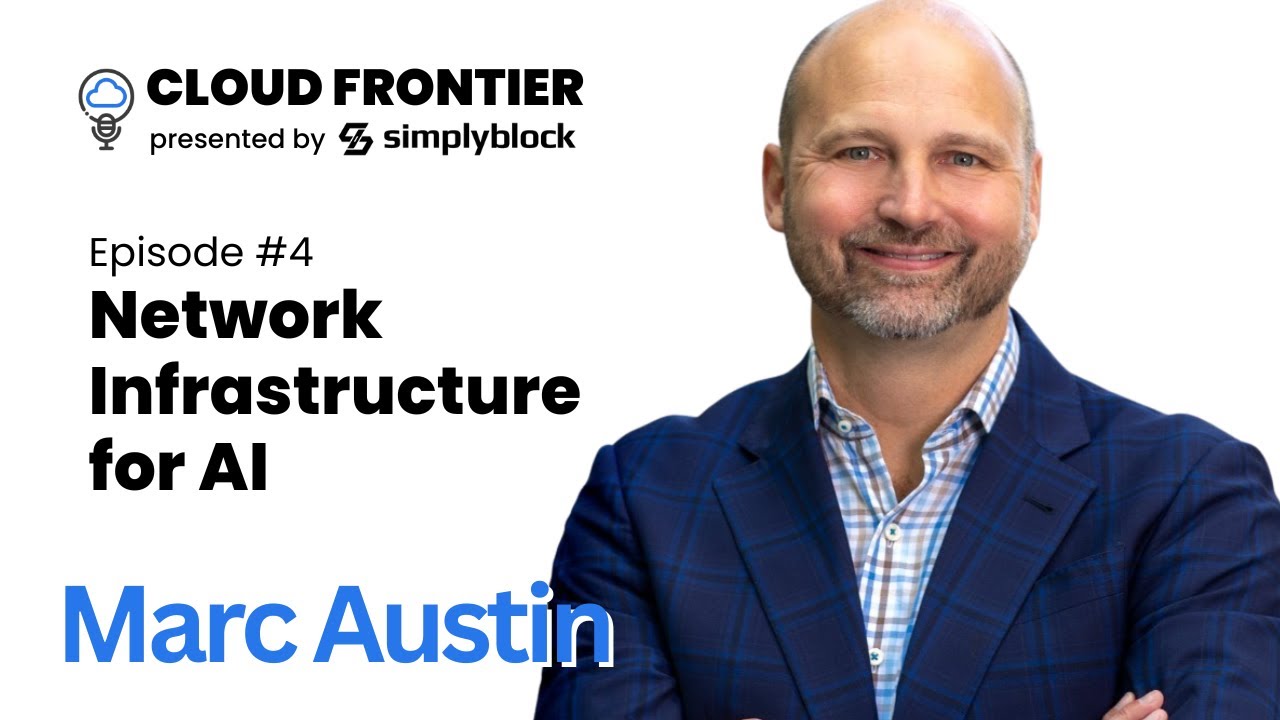
In addition to highlighting the key takeaways, it’s essential to provide context that enriches the listener’s understanding of the episode. By offering this added layer of information, we ensure that when you tune in, you’ll have a clearer grasp of the nuances behind the discussion. This approach helps shed light on the reasoning and perspective behind the thoughtful questions posed by our host, Rob Pankow. Ultimately, this allows for a more immersive and insightful listening experience.
Key Learnings
How do you Optimize Network Performance for AI Workloads?
Optimizing network performance for AI workloads involves reducing latency and ensuring high bandwidth to avoid bottlenecks in communication between GPUs. Simplyblock enhances performance by offering a multi-attach feature, which allows multiple high-availability (HA) instances to use a single volume, reducing storage demand and improving IOPS performance. This optimization is critical for AI cloud infrastructure, where job completion times are directly impacted by network efficiency.
Simplyblock Insight:
Simplyblock’s approach to optimizing network performance includes intelligent storage tiering and thin provisioning, which help reduce costs while maintaining ultra-low latency. By tiering data between fast NVMe layers and cheaper S3 storage, simplyblock ensures that hot data is readily available while cold data is stored more economically, driving down storage costs by up to 75%.
What are the Hardware Requirements for AI Cloud Infrastructure?
The hardware requirements for AI cloud infrastructure are primarily centered around GPUs, high-speed networking, and scalable storage solutions. Marc points out that AI workloads, especially for training models, rely heavily on GPU clusters to handle the large datasets involved. Ensuring low-latency connections between these GPUs is crucial to avoid delays in processing.
Simplyblock Insight:
Simplyblock addresses these hardware needs by optimizing storage performance with NVMe-oF (NVMe over Fabrics) architecture, which allows data centers to deploy high-speed, low-latency storage networks. This architecture, combined with storage tiering from NVMe to Amazon S3, ensures that AI workloads can access both fast storage for active data and cost-effective storage for archival data, optimizing resource utilization.
Additional Nugget of Information
Why is Multi-cloud Infrastructure Important for AI Workloads?
Multi-cloud infrastructure provides the flexibility to distribute AI workloads across different cloud environments, reducing reliance on a single provider and enhancing data control. For AI, this allows enterprises to run training tasks in one environment and inference at the edge, across multiple clouds. Multi-cloud strategies also prevent vendor lock-in and enable enterprises to use the best cloud services for specific workloads, enhancing both performance and cost efficiency.
Conclusion
Marc Austin’s journey with Hedgehog reveals a strong commitment to making AI network infrastructure accessible to companies of all sizes. By leveraging open-source software and focusing on distributed cloud strategies, Hedgehog is enabling organizations to run their AI workloads with the same efficiency as hyperscalers — without the excessive costs. With AI infrastructure evolving rapidly, it’s clear that companies will increasingly turn to innovative solutions like Hedgehog to optimize their networks for the future of AI.
Tune in to future Cloud Frontier Podcast episodes for insights on cloud startups, entrepreneurship, and bringing visionary ideas to market. Stay updated with expert insights that can help shape the next generation of cloud infrastructure innovations!